Since the widespread migration to the cloud, DLP has become an essential—yet often dreaded—tool for protecting data from leaks, breaches, exfiltration, and more. It’s no secret that traditional DLP solutions have a less-than-stellar reputation. Security teams are squeezed tighter than ever in terms of time and resources. Needless to say, adding more alerts on top of already daunting workloads is less than ideal. It’s time for a smarter, more sustainable form of DLP. But first, let’s take a look at the two most pressing problems that we need to solve.
Problem #1: Too many false positive alerts
Traditional DLP solutions tend to rely on low-fidelity machine learning approaches. This commonly includes:
Let’s zoom in on the first of these approaches: Regexes. While regexes’ pattern-matching capabilities come in handy from time to time, they also have their limitations. For instance, imagine that you’re trying to use a regex to scan a document for U.S. social security numbers. You direct your regex to search for nine-digit numbers, but end up getting results containing math problems, transaction numbers, and other unrelated findings.
False positive alerts like these not only clutter a security analyst’s inbox, but also interrupt data sharing that’s necessary for business workflows. While false positives can certainly be challenging to small and medium-sized businesses (SMBs), they can be even more detrimental to larger enterprises, where thousands of messages, emails, and other communications occur on a minute-to-minute basis. In this case, false positive alerts would not only take up inordinate hours of an analyst’s time, but also lead to significant workflow interruptions elsewhere in the business.
Solution #1: Advanced GenAI detection
Generative AI (GenAI) approaches, like transformer models and image classification models, excel because they have the capability to evaluate the context surrounding possible sensitive data findings.
How does this enhanced contextual understanding play out? Let’s dive into another example. This time, imagine that you work for a healthcare company, and that you’re responsible for coordinating a project to re-pave the parking lot outside your facility. In order to update your team, you send a quick Slack message including the name of the contact from the construction company, the address of your facility, and the word “cancer.”
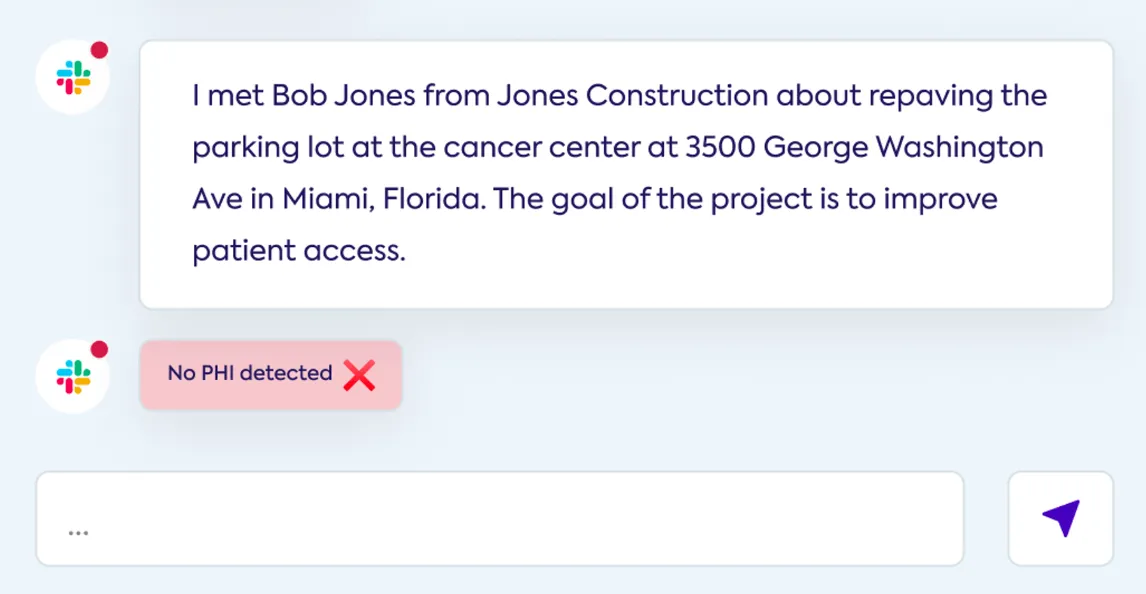
A less sophisticated detector would likely flag this message as containing PHI since it detects a possible diagnosis (”cancer”) along with an individual (”Bob Jones”). However, a GenAI-powered PHI detector like Nightfall’s would be able to leverage relevant context to determine that, in this case, “Cancer” isn’t a diagnosis, “Bob Jones” isn’t a patient, and the two entities aren’t related in any meaningful way.
HIPAA defines PHI as health data (such as an ICD-10 diagnosis description or an FDA drug name) that can be traced to a uniquely identifiable individual.
This example is just one instance when GenAI detectors can minimize false positive alerts. In Nightfall’s case, our detectors are, on average, 2x more precise than comparable detectors at competitors like Microsoft Purview, Google DLP, and AWS Comprehend. This 2x increase in precision translates to a 4x decrease in false positive alerts.

Nightfall’s detectors can also be fine-tuned with minimal feedback, leading to improved precision and lighter workloads over time. This brings us to our next point: Simplifying workflows.
Problem #2: High cost of ownership
When you combine a high volume of false positives with manual investigation and remediation, you get a very high total cost of ownership (TCO) for traditional DLP products.
If a security team is using a traditional DLP solution that has 40% rate of precision, and 60% rate of false positives, they may be so overloaded with alerts that a high-risk true positive slips through the cracks. This not only sacrifices their business’ security posture, but also puts the business at risk of privilege escalation attacks, noncompliance, or a myriad of other possible threats.
Furthermore, if a DLP solution has a high volume of false positive alerts, it’s likely not in a security team’s best interest to automate processes like remediation. This would cause a high volume of unnecessary workflow interruptions, leading to frustration and friction between the security team and non-security employees.
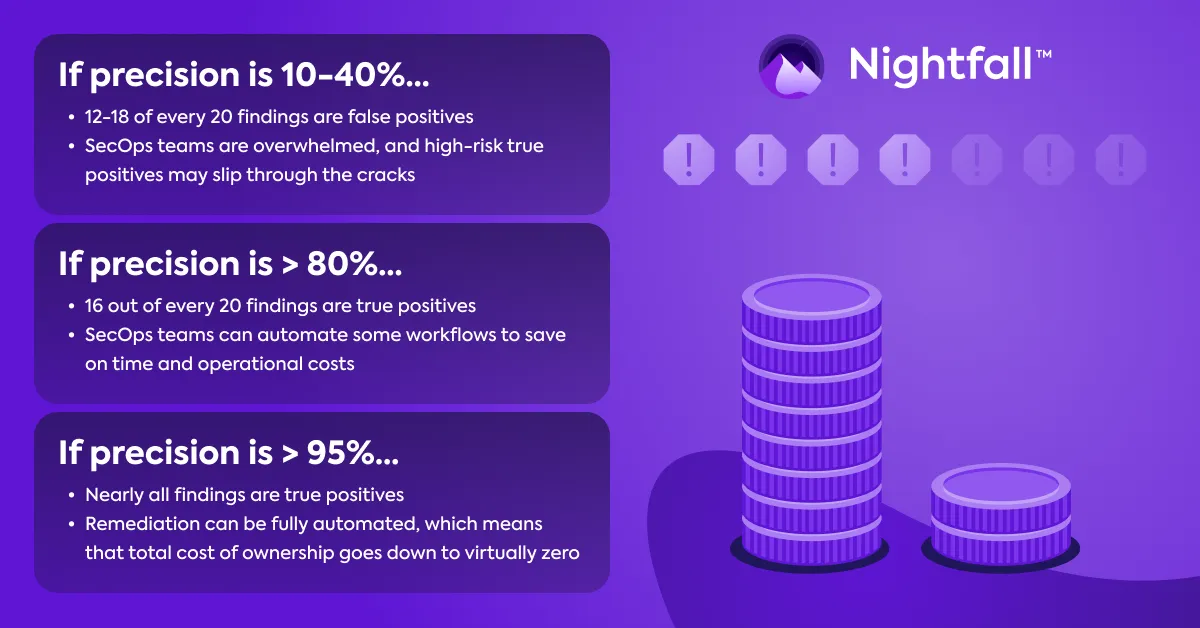
Solution #2: Automated workflows
GenAI-powered DLP solutions not only reduce the overall volume of false positive alerts, but also provide security teams with the flexibility to automate key workflows. For instance, in Nightfall’s case, that same 2x increase in precision also leads to a 4x reduction in overall cost of ownership—not including additional time savings from automation.
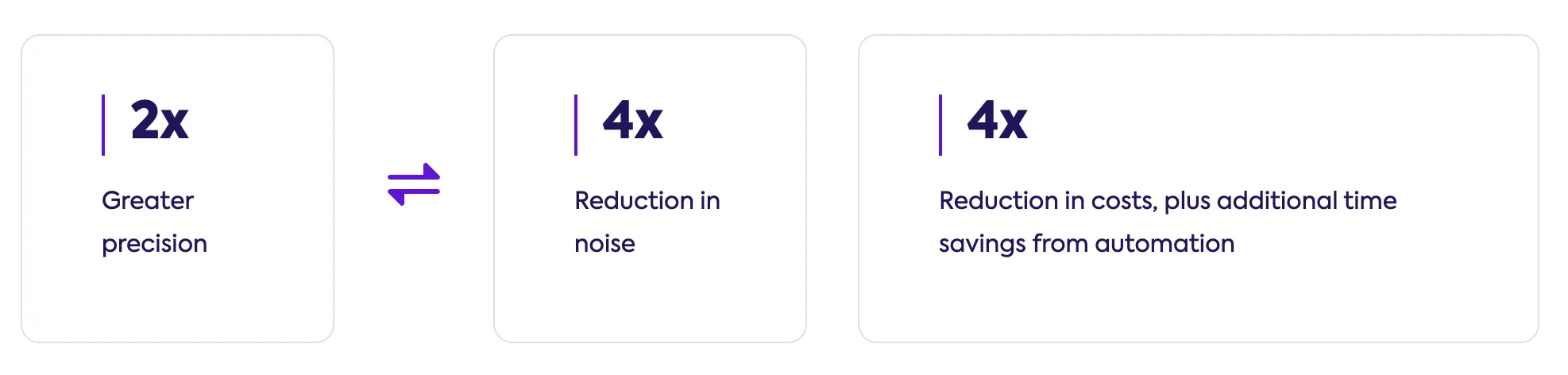
There are multiple ways in which security teams can automate their workflows, especially when it comes to investigation and remediation. Nightfall does just that with our “Human Firewall” feature. Here’s an example of the feature in action:
- An employee accidentally shares a file containing sensitive data over a popular communication app like Slack.
- In near-real time, that employee receives a custom notification that lets them know when and how they violated their business’s security policy.
- The employee has the option to automatically remediate the sensitive data in question, provide a business justification, or report a false positive alert—all without leaving the original notification.
- The employee’s response is logged instantly in the Nightfall console, eliminating the need for security teams to investigate the incident further.
The “Human Firewall” feature is an excellent example of how automation can assist security teams in the short term as well as in the long term. In the short term, security teams are able to offload alerts to employees who know their workflows better than anyone else. And in the long term, security teams are able to educate employees about company policies in hyper-specific scenarios, thereby improving their business’ security posture over time.
The TL;DR
Traditional DLP solutions often burden security teams with an abundance of false positive alerts, and offer limited opportunities for automation. However, with GenAI, it’s possible to bypass these shortcomings. GenAI-powered DLP solutions not only offer increased precision, but also more seamless workflows for both security and non-security employees.
GenAI is a crucial part of Nightfall’s vision: To create a future where DLP is a smarter, more efficient, and more scalable solution for businesses big and small.